Neural networks (NN) have become a cornerstone of modern artificial intelligence (AI) and machine learning (ML). Whether you're a developer, researcher, or simply someone fascinated by technology, understanding neural networks is essential for grasping how machines mimic human-like intelligence. NNs are algorithms designed to recognize patterns and learn from data, much like the human brain processes information. This article will explore the intricacies of neural networks, their applications, and how they are transforming industries worldwide.
In recent years, neural networks have gained prominence due to their ability to solve complex problems that traditional algorithms cannot handle. From image recognition to natural language processing, NNs have proven their versatility and effectiveness. As AI continues to evolve, understanding these networks becomes increasingly important for anyone interested in staying ahead of the technological curve.
This comprehensive guide will take you on a journey through the world of neural networks, breaking down complex concepts into digestible information. Whether you're a beginner or an advanced learner, this article aims to provide valuable insights into the mechanics, applications, and future potential of NNs.
Read also:Top 10 Funniest Nba Names A Laughoutloud Journey Through Basketball History
Table of Contents
- What is a Neural Network?
- History of Neural Networks
- Components of a Neural Network
- Types of Neural Networks
- Applications of Neural Networks
- Advantages and Challenges of Neural Networks
- Training Neural Networks
- The Future of Neural Networks
- Real-World Examples of Neural Networks in Action
- Conclusion
What is a Neural Network?
Neural networks are computational models inspired by the structure and function of the human brain. These networks consist of layers of interconnected nodes, or "neurons," that process input data and produce outputs. At their core, neural networks are designed to identify patterns and relationships within datasets, enabling them to make predictions or decisions based on that data.
Key Characteristics of Neural Networks
Neural networks possess several defining characteristics that set them apart from other algorithms:
- Learning Capability: Neural networks can learn from data, improving their accuracy over time.
- Adaptability: They adapt to new information, making them suitable for dynamic environments.
- Parallel Processing: Neural networks can process multiple inputs simultaneously, enhancing efficiency.
These features make neural networks ideal for solving complex problems in various fields, including healthcare, finance, and autonomous systems.
History of Neural Networks
The concept of neural networks dates back to the mid-20th century, with the development of the first artificial neuron model by Warren McCulloch and Walter Pitts in 1943. Since then, the field has undergone significant evolution, driven by advances in computing power and data availability.
Key Milestones in Neural Network Development
- 1950s-1960s: The Perceptron, one of the earliest neural network models, was introduced by Frank Rosenblatt in 1958.
- 1980s: The backpropagation algorithm, crucial for training deep neural networks, was developed.
- 2010s: The rise of deep learning, fueled by advancements in GPU technology and big data, revolutionized neural network applications.
Today, neural networks are at the forefront of AI innovation, powering technologies such as self-driving cars, speech recognition systems, and personalized recommendation engines.
Components of a Neural Network
A neural network comprises several key components that work together to process and analyze data:
Read also:Bbe Chsn The Rising Star In The Music Industry
1. Neurons
Neurons are the basic building blocks of a neural network. Each neuron receives input, processes it, and produces an output that is passed to the next layer. The activation function determines how the neuron responds to its input.
2. Layers
Neural networks are organized into layers, each serving a specific purpose:
- Input Layer: Receives raw data as input.
- Hidden Layers: Process the data and extract features.
- Output Layer: Produces the final output or prediction.
3. Weights and Biases
Weights and biases are adjustable parameters that influence how data flows through the network. During training, these parameters are optimized to minimize errors and improve performance.
Types of Neural Networks
There are various types of neural networks, each suited to different tasks:
1. Feedforward Neural Networks
Feedforward networks are the simplest type, where data flows in one direction, from input to output. They are commonly used for classification and regression tasks.
2. Convolutional Neural Networks (CNNs)
CNNs are specialized for image and video processing, leveraging convolutional layers to detect spatial patterns.
3. Recurrent Neural Networks (RNNs)
RNNs are designed for sequential data, such as time series or natural language, allowing them to retain information across time steps.
4. Deep Belief Networks (DBNs)
DBNs are generative models that combine multiple layers of latent variables, enabling unsupervised learning.
Applications of Neural Networks
Neural networks have a wide range of applications across industries:
1. Healthcare
In healthcare, neural networks are used for medical imaging analysis, disease diagnosis, and drug discovery. For example, CNNs can detect anomalies in X-rays or MRIs with remarkable accuracy.
2. Finance
Financial institutions employ neural networks for fraud detection, credit scoring, and stock market prediction. These models analyze vast amounts of data to identify patterns and make informed decisions.
3. Autonomous Systems
Self-driving cars rely on neural networks for object detection, path planning, and decision-making. These networks process sensor data in real-time to ensure safe and efficient operation.
Advantages and Challenges of Neural Networks
While neural networks offer numerous benefits, they also present certain challenges:
Advantages
- High Accuracy: Neural networks can achieve superior performance on complex tasks.
- Scalability: They can handle large datasets and high-dimensional data.
- Adaptability: Neural networks can learn from new data and improve over time.
Challenges
- Computational Complexity: Training deep neural networks requires significant computational resources.
- Data Dependency: Neural networks often require large amounts of labeled data for effective training.
- Interpretability: Understanding how neural networks arrive at their decisions can be challenging.
Training Neural Networks
Training a neural network involves optimizing its parameters to minimize errors between predicted and actual outputs. This process typically follows these steps:
1. Data Preparation
Data must be preprocessed, normalized, and split into training, validation, and test sets to ensure reliable results.
2. Model Architecture
Selecting the appropriate architecture depends on the problem at hand. Factors such as network depth, layer types, and activation functions must be carefully considered.
3. Optimization
Optimization algorithms, such as stochastic gradient descent (SGD), adjust the network's weights to reduce loss during training.
The Future of Neural Networks
As technology continues to advance, the future of neural networks looks promising. Emerging trends include:
1. Explainable AI
Researchers are developing methods to enhance the interpretability of neural networks, making their decisions more transparent and trustworthy.
2. Edge Computing
Deploying neural networks on edge devices enables real-time processing and reduces latency, making them ideal for applications like IoT and robotics.
3. Hybrid Models
Combining neural networks with other machine learning techniques can improve performance and address limitations of individual approaches.
Real-World Examples of Neural Networks in Action
Neural networks are already transforming industries worldwide. Some notable examples include:
1. AlphaGo
Developed by DeepMind, AlphaGo defeated the world champion in the game of Go, showcasing the power of reinforcement learning and neural networks.
2. Tesla Autopilot
Tesla's self-driving system relies on neural networks to process sensor data and make driving decisions, enhancing safety and convenience.
3. Google Translate
Google's neural machine translation system provides accurate and fluent translations across multiple languages, revolutionizing communication.
Conclusion
Neural networks have become indispensable tools in the field of artificial intelligence, driving innovation and solving complex problems. From their humble beginnings to their current state-of-the-art capabilities, neural networks continue to evolve and expand their reach.
In conclusion, understanding neural networks is crucial for anyone interested in AI and machine learning. By exploring their components, applications, and future potential, we can appreciate their significance in shaping the world we live in. We encourage you to share your thoughts, ask questions, or explore related articles to deepen your knowledge further.
Let us know what you think in the comments below, and don't forget to share this article with others who might find it valuable!
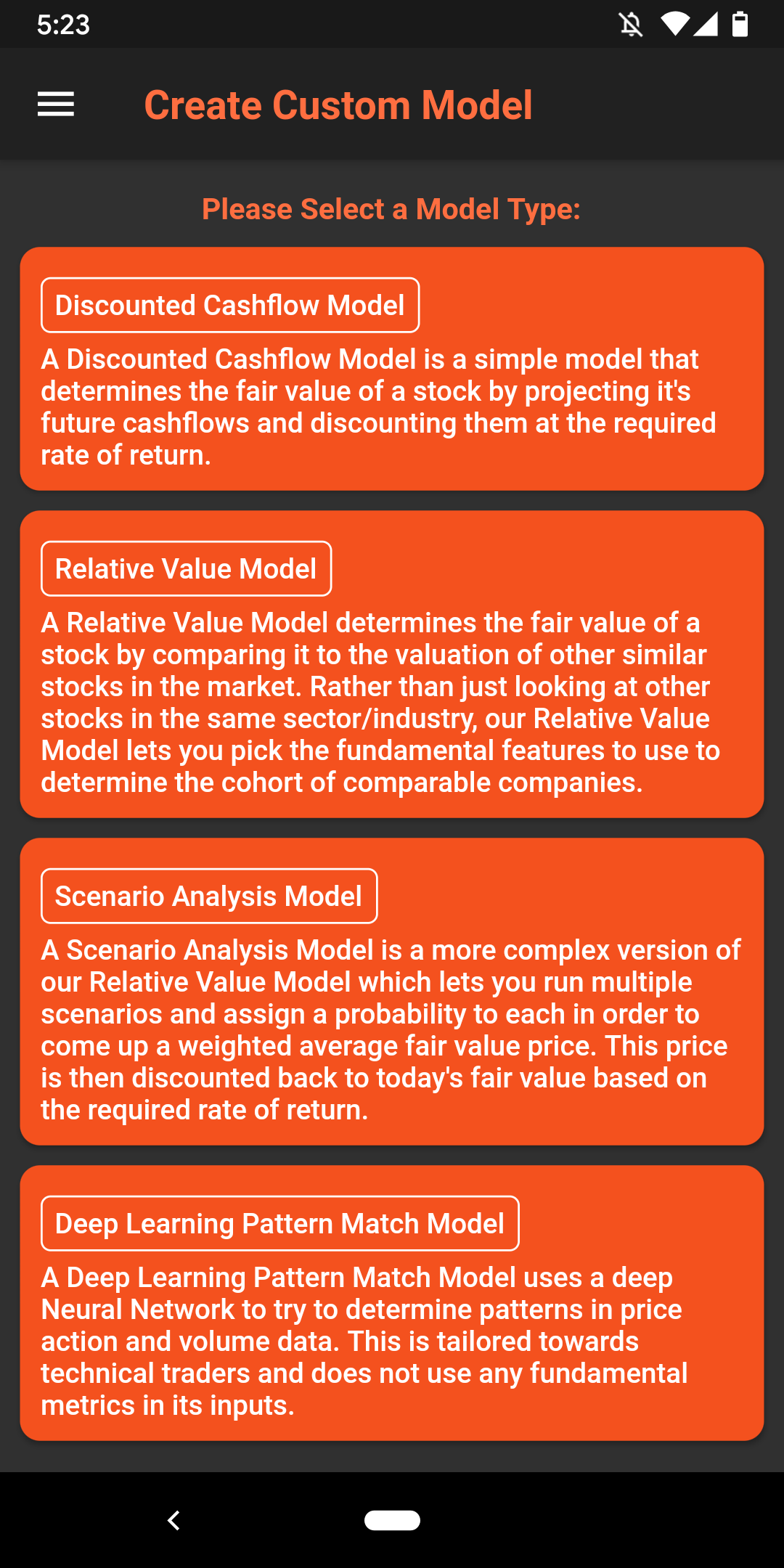
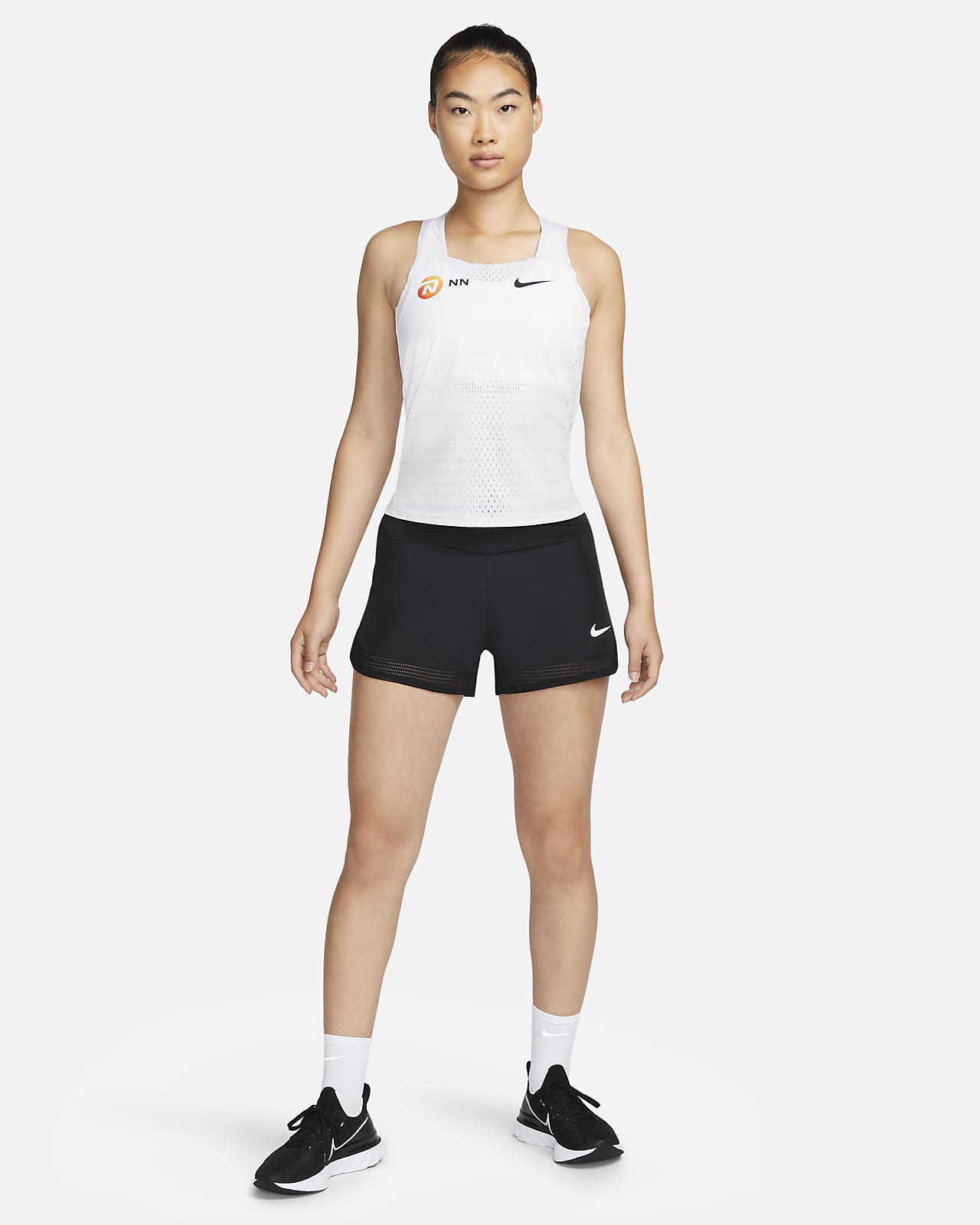
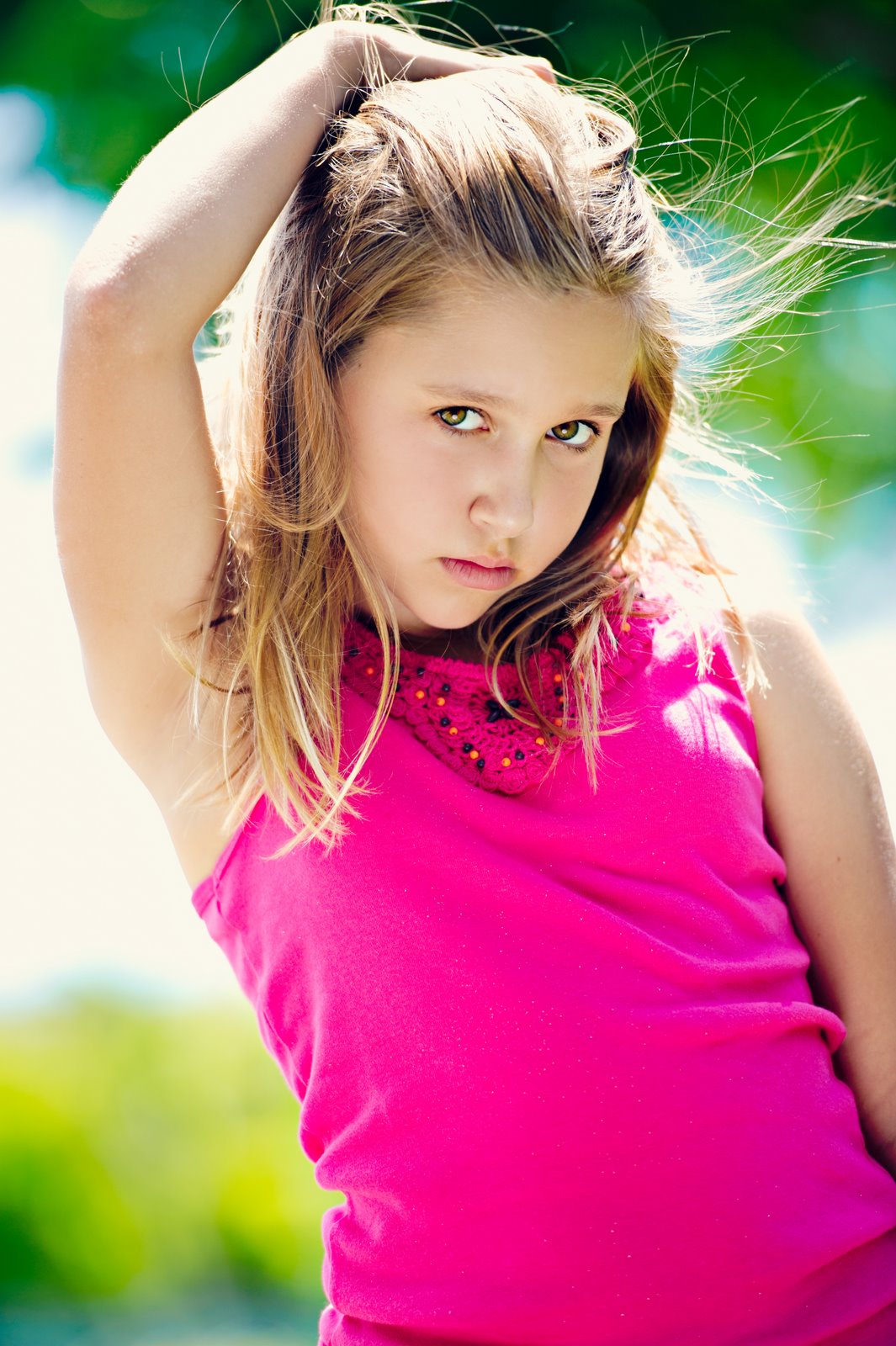